This post introduces a Python library for estimation of the dynamic Nelson-Siegel (DNS).
Estimation and Forecast of Dynamic Nelson-Siegel model
I found the following useful Python library for estimating and forecasting the DNS or Svensson (DNSS) model.
In the Jupyter notebook, this library can be installed by running the following command.
!pip install Dynamic-Nelson-Siegel-Svensson-Kalman-Filter
Correlated-factor DNS model
This Python DNS library use the specification of correlated-factor DNS model instead of the independent-factor model more generally. The correlated-factor DNS model can be expressed as the state space representation which consists of both measurement and state equation as follows.
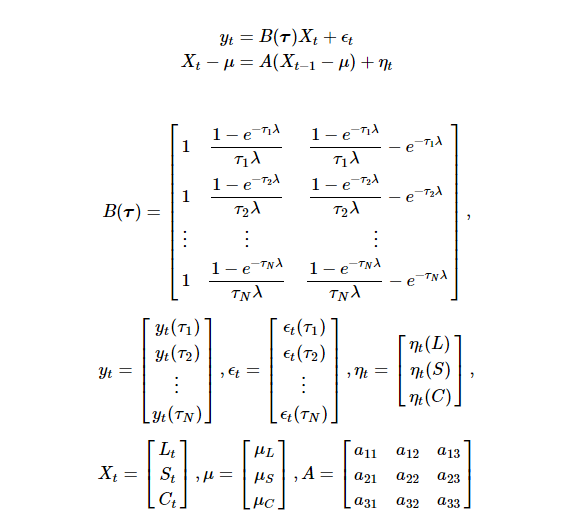
where yt(τ) is continuously compounded spot rates of maturity τ at time t. Lt,St,Ct are level, slope, curvature factors respectively and its unconditional means and autoregressive coefficients are denoted as μL,μS,μC and ϕL,ϕS,ϕC sequentially. λ is an exponential time decay parameter. ϵt follows a multivariate normal distribution with only diagonal variances, in other words, a diagonal covariance matrix.
Taking correlated-factors into account, ηt has a non-diagonal variance-covariance matrix ???? = ???? × ????.

Python Jupyter Notebook Code
From the web page above, sample data can be obtained, which is the Diebold paper’s monthly U.S yield curve dataset.
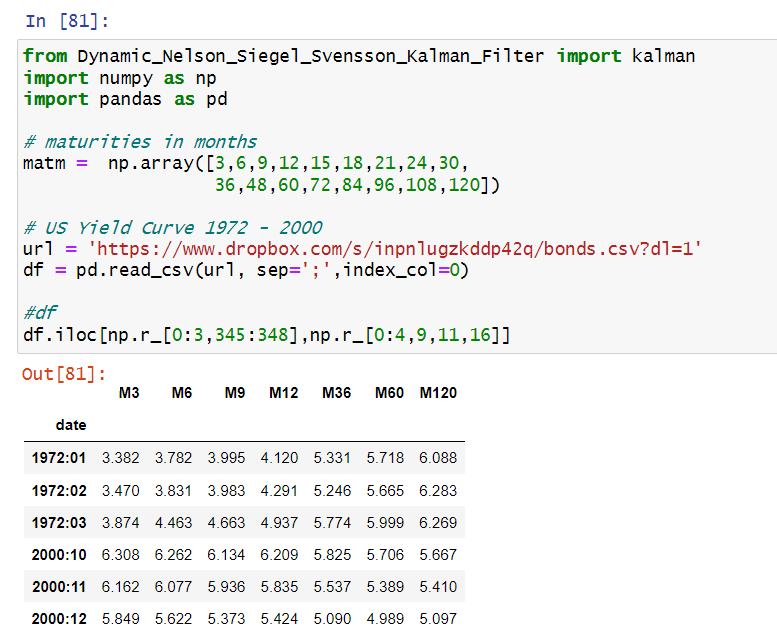
Initial guesses for parameters are prepared and this is a rounded version of initial guesses which are provided by the above web page. I redefine an objective function (objf) based on kalman() function in this library to reduce the number of arguments to be passed. I also define a callback function (callbackF) which prints function evaluations periodically to check the progress of this optimization.
The dynamic Nelson-Siegel-Svensson (DNSS) model can also be estimated by setting model = ‘S’.
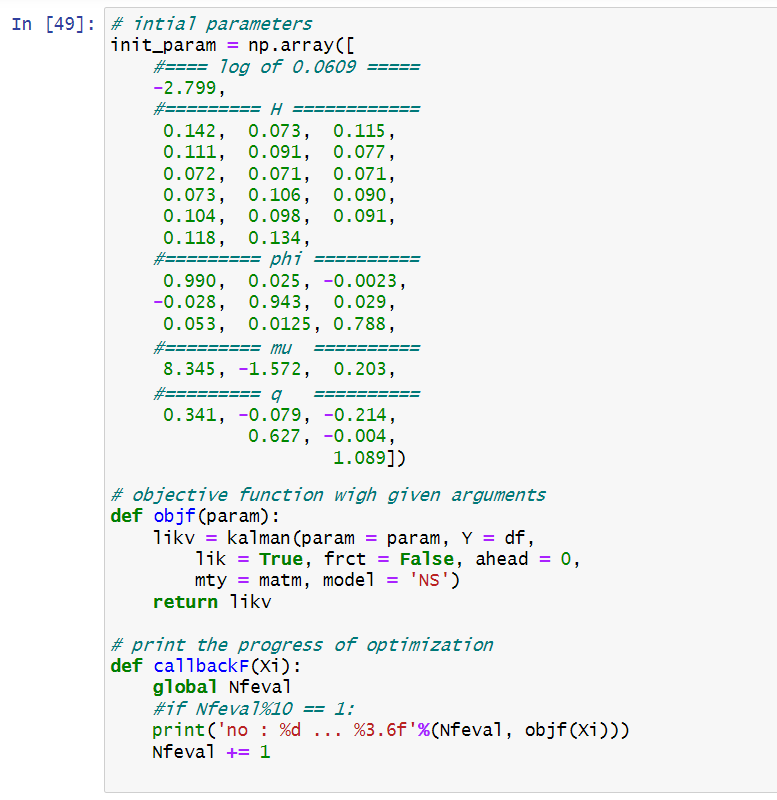
Optimization is done by using optimize.minimize() function of scipy library with ‘L-BFGS-B‘ algorithm. Of course, there are many alternative optimization algorithm such as ‘Nelder-Mead‘, ‘BFGS‘, and ‘CG‘ to name a few. The callback function prints the number of iteration and the function evaluations.
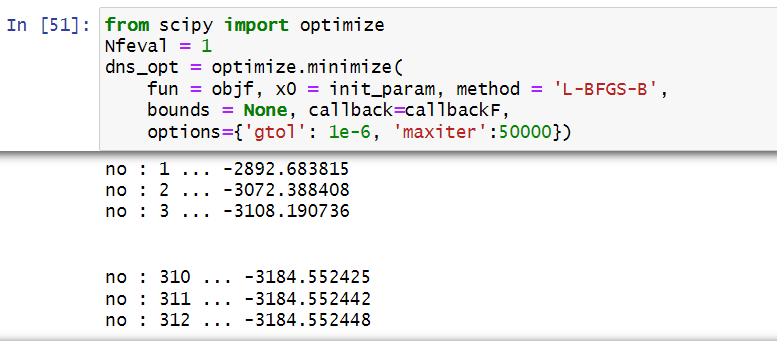
After many iterations, we can get the following results of estimation.
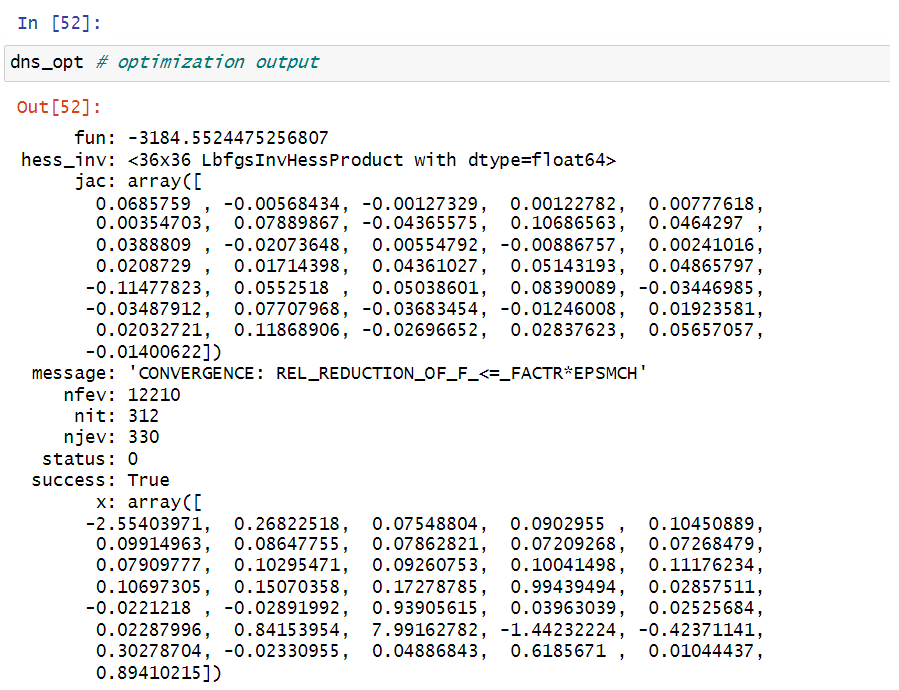
Parameter estimates are stored at x of the resulted object (dns_opt.x).
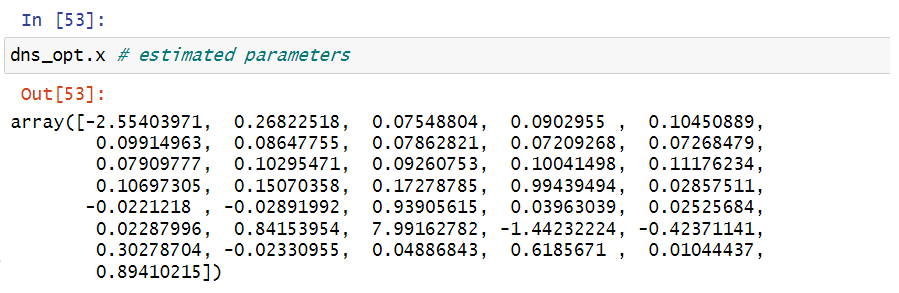
Concluding Remarks
This post gives a guided-tour of how to use a Python library for estimation of dynamic Nelson-Siegel model. This also can be useful when the dynamic Svensson model needs to be estimated.
Originally posted on SHLee AI Financial Model blog.
Disclosure: Interactive Brokers Third Party
Information posted on IBKR Campus that is provided by third-parties does NOT constitute a recommendation that you should contract for the services of that third party. Third-party participants who contribute to IBKR Campus are independent of Interactive Brokers and Interactive Brokers does not make any representations or warranties concerning the services offered, their past or future performance, or the accuracy of the information provided by the third party. Past performance is no guarantee of future results.
This material is from SHLee AI Financial Model and is being posted with its permission. The views expressed in this material are solely those of the author and/or SHLee AI Financial Model and Interactive Brokers is not endorsing or recommending any investment or trading discussed in the material. This material is not and should not be construed as an offer to buy or sell any security. It should not be construed as research or investment advice or a recommendation to buy, sell or hold any security or commodity. This material does not and is not intended to take into account the particular financial conditions, investment objectives or requirements of individual customers. Before acting on this material, you should consider whether it is suitable for your particular circumstances and, as necessary, seek professional advice.
Join The Conversation
For specific platform feedback and suggestions, please submit it directly to our team using these instructions.
If you have an account-specific question or concern, please reach out to Client Services.
We encourage you to look through our FAQs before posting. Your question may already be covered!