It is not easy to build a robust trend-following strategy that will withstand different difficult market conditions and bring consistent results. The author of today’s work was not frightened by this task and delivered a full framework on how to design a robust trend-following strategy step by step.
The method presents sensitivity analysis and robustness checks through various time horizons and sample choices. It also accounts for transaction costs (when one rebalances often, they creep in and eat out a significant chunk of profits) and takes a multi-asset approach to maximize closely watched risk metrics from PMs (portfolio managers).
Dobromir Tzotchev’s framework can be summarized into the following steps:
- Need for a clean Trend-following Signal: The paper proposes a trend-following time-series momentum signal based on statistical theory and investigates its properties. It reconciles theoretical results with stylized facts about trend-following investing, including the link to straddles and the better performance of so-called “slower” signals.
- Sound Design and Prototype Solution: Based on theoretical results, the paper introduces a prototype trend-following solution that uses a unified approach across assets and diversifies across time frames. Through simulation examples, it highlights performance versus benchmarks and diversification properties for long-only portfolios.
- Risk Management Techniques: The paper elaborates on portfolio and risk management for trend-following strategies. It adapts risk-budgeting and Hierarchical Risk Parity (HRP) approaches to the trend-following framework. Additionally, it discusses methods to manage transaction costs and implications of the carry component in futures and FX forwards.
Authors: Dobromir Tzotchev
Title: Designing Robust Trend-following System: Behind the Scenes of Trend-following
Link: https://papers.ssrn.com/sol3/papers.cfm?abstract_id=4677166
Abstract:
Trend-following has actively been on investors’ radar for the last few decades. The J.P. Morgan primer on momentum strategies (Kolanovic and Wei, 2015) provides an extensive review of the momentum strategies. The current paper focuses on a concrete trend-following solution and analyzes its properties alongside the practical implementation.
As always we present several interesting figures and tables:
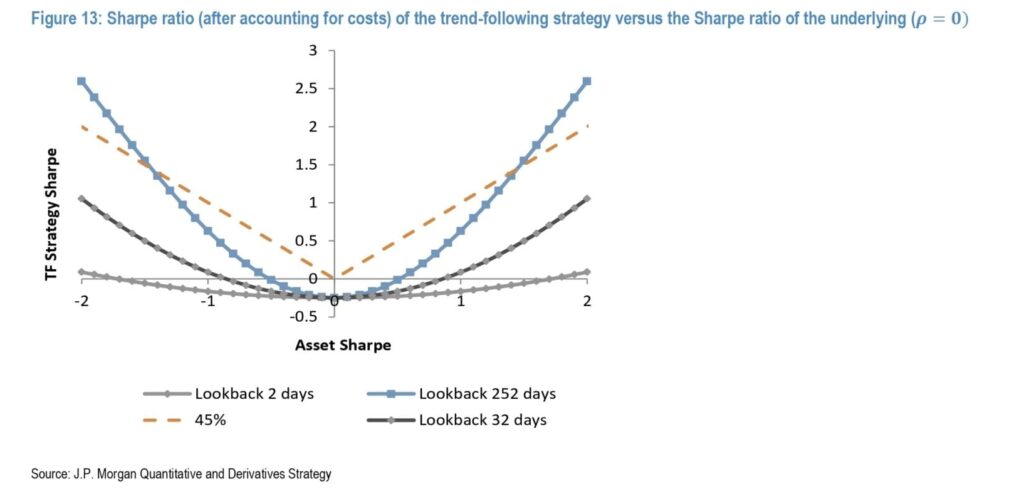
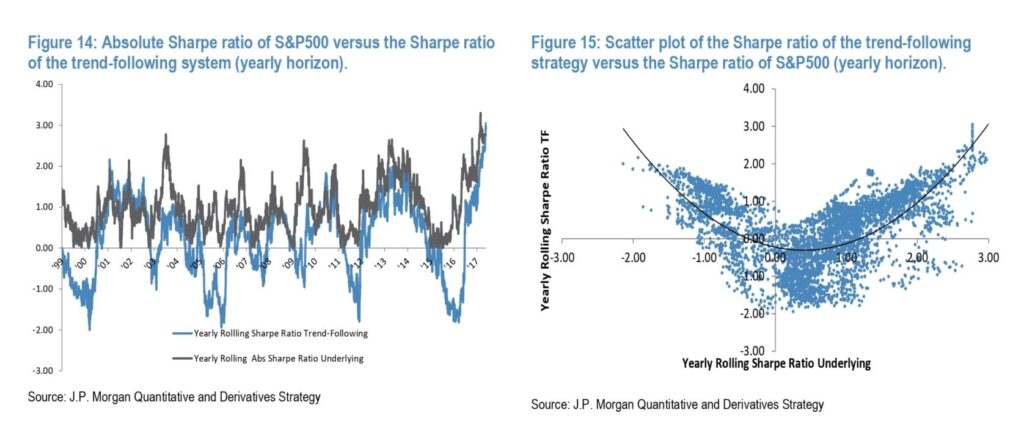
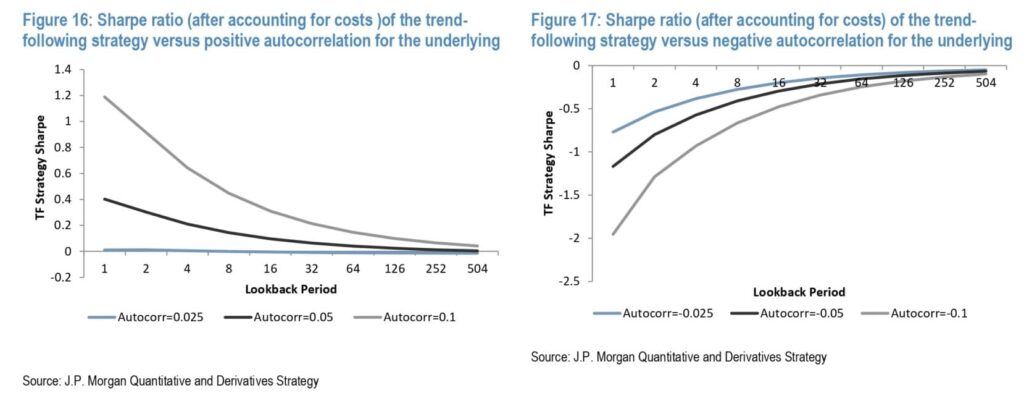
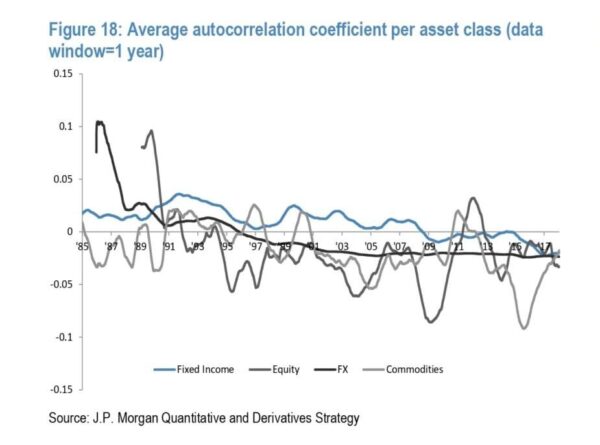
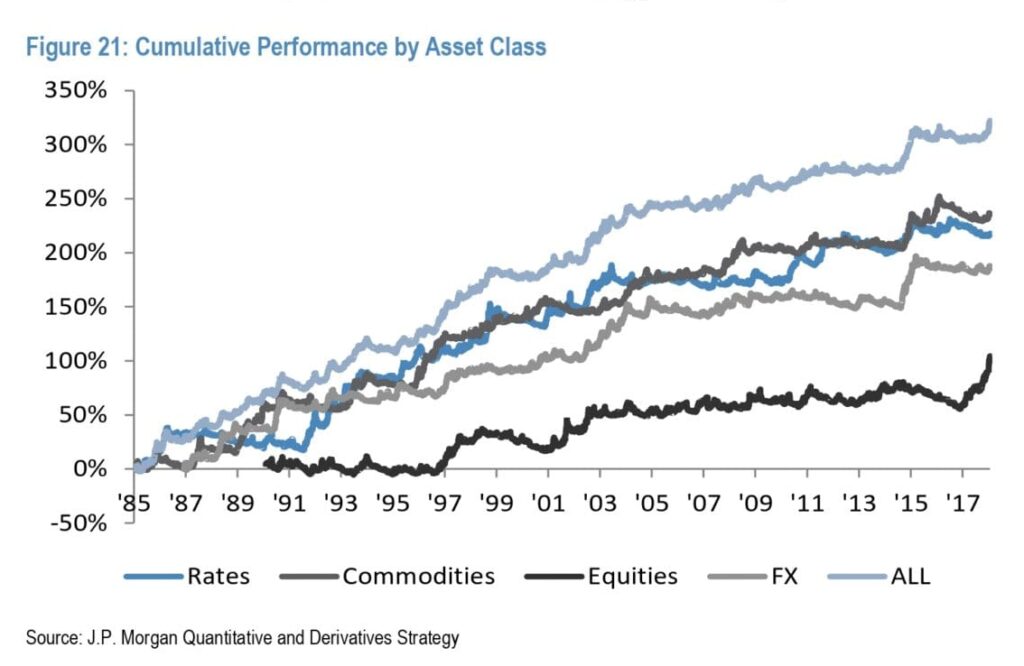
Notable quotations from the academic research paper:
“We start by presenting a signal that is based on statistical hypothesis testing. We show that under certain conditions the trend-following signal is the also the delta of a straddle. Hence we make explicit the widely propagated link between trend- following and long straddle positions (see for example Fung and Hsieh 2011).
Subsequently, we analyze the profit drivers for the trend-following strategy based on the proposed signal. We show that the strategy (similarly to a straddle) is profitable whenever there are trends in either direction. Hence we demonstrate that the so-called “CTA smile” (see for example Hurst et. al. 2014) can be justified within a theoretical model as well. Furthermore, the strategy exhibits convexity. The absolute value of the Sharpe ratio of the underlying asset is of critical importance for the profitability of the strategy and the higher the number, the bigger the convexity embedded in the strategy. Furthermore, signals based on longer estimation periods possess ceteris paribus better profitability than signals based on shorter lookback periods.
Next, the time-series properties of the underlying asset are explicitly taken into account. We show that the autocorrelation is important only for the profitability of signals based on short lookback periods (typically less than a month). Naturally positive autocorrelation leads to profits while even small values of negative autocorrelation induce substantial losses. On the other hand the profitability of the signals based on longer lookback periods is unaffected by the time-series properties of the underlying.
Due to the non-linear nature of the expressions for the expected P&L and transaction costs, it is difficult to derive the threshold Sharpe ratio of the underlying that renders the profitability of a signal based on a certain lookback period. Nevertheless, numerical results shed some interesting caveats for this relationship. In Figure 13 we have plotted the Sharpe ratio based on the net P&L of the trend-following strategy versus the Sharpe ratio of the underlying for various lookback periods. We use the transaction cost structure for S&P and assume a daily volatility of 1% (approximately 16% annualized). It is evident that signals based on short term lookbacks can only be profitable if the Sharpe ratio of the asset is quite sizable in either direction. For example, for a signal based on 2 days we need a Sharpe ratio above 2 and below -2 to assure the profitability of the strategy. For a signal based on 32 days, the Sharpe ratio should be above 1 or below -1. Even a signal based on a 1 year lookback period requires the absolute value of the Sharpe ratio to be bigger than 0.5 so that profitability is assured.
Furthermore, we expect the Sharpe ratio of trend-following strategy to be below the absolute value of the Sharpe ratio of the asset. A sizable positive or negative Sharpe ratio of the underlying and long term lookback period are both necessary for the Sharpe ratio of the trend-following strategy to exceed the absolute value of the Sharpe of the underlying. For example, we need the Sharpe ratio of the underlying to be bigger in absolute value than 1.5 so that trend-following is more profitable than either holding or shorting the asset.
If the drift of the asset is stable (stays constant over a long period), it is much more profitable and cost-efficient to use signals based on longer lookback periods. For example, if we expect equities to exhibit a positive drift due to the embedded equity risk premia, it is preferable to use signals with longer lookback periods. The appeal of the shorter term lookback periods arises in two scenarios. Firstly, the duration of the trend might be smaller than a long lookback period. For example, if the trend changes direction every 6 months making use of a signal based on 1 year lookback will be detrimental. Secondly, during market reversals signals based on shorter lookback periods are more reactive and eventually mitigate the drawdowns of the slower trend-following systems.
[On Figure 21: Cumulative Performance by Asset Class] the cumulative performance of the benchmark approach in various asset classes as well as the performance of the combined portfolio are shown14. Commodities have historically had the most appealing trend-following track-record (commodities are also the asset class upon which the CTA industry originated). The asset class that has been historically been the most challenging for the trend-following approach is equities.
In addition to the attractive feature of positive skewness that the trend-following strategies possess, trend-following strategies bring substantial diversification benefits for the long-only portfolios. As we have already shown in the theoretical sections, trend-following strategies exhibit convexity and when the move on the downside is sizable enough the return of the trend-following strategy will more than compensate the loss in the underlying. It has also been well-known that the magnitude of the sell-offs is typically quite sizable and therefore the offset with the trend-following strategies is quite appealing.
To verify this hypothesis empirically we have constructed portfolios that consist of long positions in the underlyings from our asset universe. The portfolios are well targeted to have an annualized volatility of 10% and utilize the same risk weights for the individual assets as in our benchmark solution. We have also constructed combined portfolios that invest 50% in the long-only portfolio and 50% in the trend-following system. The diversification benefits are quite evident in all asset classes except for fixed income. In fixed income, the directionality of the market has led to a lot of overlap between the positions of the trend-following system and those of the long-only portfolio.”
Originally posted on Quantpedia.
Disclosure: Interactive Brokers Third Party
Information posted on IBKR Campus that is provided by third-parties does NOT constitute a recommendation that you should contract for the services of that third party. Third-party participants who contribute to IBKR Campus are independent of Interactive Brokers and Interactive Brokers does not make any representations or warranties concerning the services offered, their past or future performance, or the accuracy of the information provided by the third party. Past performance is no guarantee of future results.
This material is from Quantpedia and is being posted with its permission. The views expressed in this material are solely those of the author and/or Quantpedia and Interactive Brokers is not endorsing or recommending any investment or trading discussed in the material. This material is not and should not be construed as an offer to buy or sell any security. It should not be construed as research or investment advice or a recommendation to buy, sell or hold any security or commodity. This material does not and is not intended to take into account the particular financial conditions, investment objectives or requirements of individual customers. Before acting on this material, you should consider whether it is suitable for your particular circumstances and, as necessary, seek professional advice.
Disclosure: Forex
There is a substantial risk of loss in foreign exchange trading. The settlement date of foreign exchange trades can vary due to time zone differences and bank holidays. When trading across foreign exchange markets, this may necessitate borrowing funds to settle foreign exchange trades. The interest rate on borrowed funds must be considered when computing the cost of trades across multiple markets.
Join The Conversation
For specific platform feedback and suggestions, please submit it directly to our team using these instructions.
If you have an account-specific question or concern, please reach out to Client Services.
We encourage you to look through our FAQs before posting. Your question may already be covered!