QMIT by QuantZ presents Q1 2020 Factor recap. To learn more about QuantZ/ QMIT and to get their factor research + heatmaps daily or even real-time, please get in touch!
The Q1 Covid Crash of 2020 was tremendous live validation of our REGIME BASED FACTOR INVESTING framework with Risk OFF factors at +17.5% on avg vs Risk ON at -5.8% resulting in a +23.3% spread YTD. Except for 2 out of 18 ESBs (Deep Value & Efficiency) the other 16 beat the SPX by a wide margin in Q1 2020. If one had the right view on the regime as is required of the Quantamental hybrid discipline (& it didn’t take much rocket surgery to figure out that Covid was going to be Risk-Off after China & Italy) then it was possible to monetize much of the predictable factor dispersion which most managers still failed to do. There has been much ink spilled over the inability of quants to thrive in this higher vol/ higher dispersion environment given that many prominent managers have underperformed. At least for factor quants (as well as stat arb in general), we believe this is largely due to the failure to discern the regime dependence & cyclicality of factors. Being on the wrong side of regime based tilts or allowing your factor payoffs to effectively cancel out due to RORO is a travesty that can be avoided.
- 9 out of 11 Risk OFF ESBs were up substantially in Q1 on a $ neutral basis with the avg across all 9 being +17.5%. The lowest at +8% was Leverage while Risk [aka Low Vol] led the pack at +44% (327% ann). 4 out of 7 Risk ON ESBs were down with the avg across all 7 being -5.8% which is to be expected in a bear market showing the outsample validity of our regime mapping.
- Amongst Risk OFF ESBs, only DIV was down substantially at -10% (EQ was at -3%) & that’s because almost half of the universe does not pay dividends hence not much discernible alpha on the short side. Making matters worse, some of the highest payers on the long side come under fire in a crisis due to the likelihood of a cut just as we saw in 2008.
- On the plus side of the ledger, we saw staggering +44% returns from Risk [aka Low Vol] – on top of +20% last year – due to the obvious net beta mismatch as well as Profitability at +38% (clearly being Profitable paid off) & Stability at +31% (clearly stability of earnings, revenues, cashflows etc pays off in a crisis).
- The Momentum cohort (PMOM, ENMOM, ARS and ART) which had a good 2019 continued to pay off handsomely (PMOM +21%, EnMom +20%, ARS +21%, ART +16%) as consistent with the crash of 2008 & 2011 (hence our Risk OFF classification for such ESBs). During market turmoil the short side of momentum tends to deliver (in this case some of the Energy & Financial longs in Value). See Figure 1.
- Amongst Risk ON ESBs, DV (Deep Value) was down substantially at -27% which is to be expected during the crash phase. Interestingly, RV (Relative Value) held up a lot better at only -2.6%. Size at -14% was another classic tell of the risk aversion expressed via the market’s preference for Large over Small. Short Interest (SIRF) chipped in with +6% YTD while Growth worked at +7% YTD. See Figure 2.
- Reversals (REV) performed consistently throughout 2019 & came screaming back +24% in March after being down -40% (an ~+60% run in just 10TDs) to finish Q1 at +17.5% since shorter term reversals worked well during the historic choppiness of March.
- Most factors & the market overall was subdued till 2/19 after which the dispersion really took off in concert with vol as the Covid death toll in Italy became front page news.
- March was particularly poignant validation of our RORO regime based approach given that we saw the fastest crash in the SPX’s history (it came back from -30.5% to end Q1 at -20%) & the corresponding outsized factor moves as per heatmaps below.
- In general, ESBs perform far better on a Beta-neutral basis in Bull markets while in Bear markets it’s better to hedge on a $-neutral basis. For the most part daily rebalancing of factors helps except in such choppy markets (with the VIX at all-time highs) where the month end factor ranks actually end up outperforming in certain instances because daily rebalancing can get whipsawed.
- Figure 3 shows the rationale for our ESB regime classification.
- Early in Q1 it looked like the Value-Momentum spread was ripe for a reversal but then the Covid crash dynamic took over & further exacerbated the divergence for obvious reasons as cheap stocks got cheaper for a reason while investors continued to hide in the intersection set of Quality + Momentum. Value & Efficiency continued to plummet as an extension of 2019 just like the continued dominance of Large cap Growth (LCG) over Small cap Value (SCV). Clearly that exacerbated Value-Momentum spread will see a vicious turnaround just like in Mar of 2009 once we decisively form a bottom. Early signs of the curve flattening in NY have led to a rally but tremendous uncertainty lingers about how the world will adjust to the new realities of social distancing & get back to work.
- ESB clusters (e.g., PMOM, ENMOM, ARS and ART) correlated in terms of c-s ranks (full alpha list) are even more pronounced in terms of 3 & 20y returns correlations (based only on the 5%tile tails).
- As is characteristic of crash episodes – pairwise stock correlations spiked – diminishing the opportunity for stock selection while beta & factor risk dominated. Recall 2011 & 2008.
- Sector ranks based on the aggregation of our ESBs have been shuffled substantially post-crash which is why it’s no surprise that defensives (low vol) sectors like Utilities, Staples & Healthcare are now at the top based on PMOM, ARS, ART & Risk while the higher beta cyclicals like Energy, Materials, Discretionary & Industrials are at the bottom. The discrepancies between PMOM & ENMOM are noteworthy because they will drive the relative outperformance of ENMOM at some point. Sector ranks are negatively correlated across the Value-Mom clusters as one might expect.
- Both the long & short sides contributed to the double spread alpha in the best performing ESBs like Risk & Profitability.
- Looking out to Q2, much uncertainty pervades the outlook as market pundits & their crystal balls have been fogged up by the mist of the Coronavirus in the air. No one really knows the timeline for a return to any semblance of normalcy hence it’s difficult to divine directionality and the corresponding factor outcomes. Till there is either a vaccine or herd immunity, it’s clear that we are looking at substantial social distancing disruptions to entire sectors of the economy like restaurants, airlines, leisure etc which will likely drive unemployment to levels beyond what was seen in the Great Depression. For now one must remain tilted towards Risk Off factors but remain extremely nimble once we see the Covid curves flatten & the market starts anticipating green shoots of normalcy interjected by possible waves of recurrent infections.



The Sector ranks table (based on bottom up aggregation of QMIT Enhanced Smart Betas within sectors) allows for sector rotation based on factors. The cross-sectional factor rank correlations tell us how correlated the factors are at this juncture vs recent 3y return correlations vs LTD (20y) return correlations. It’s worth noting that cross sectional factor rank correlations are based on today’s alphas across the entire universe while the historical return correlations are only based on the information in the tails (i.e., the 5%-tile spread returns). Further, as the astute may surmise, one can extract a risk model from our factor covariance matrix which should better align one’s alphas with the risk optimization.
- Equal Weighted
- Max Sharpe Ratio optimization (on an expanding window to prevent look ahead bias)
- Risk Parity optimization (on an expanding window to prevent look ahead bias)
- Top 3 factors based on cumulative return but Equal Weighted (on an expanding window to prevent look ahead bias)
- Top 3 factors based on Sharpe ratio but Equal Weighted (based on cumulative return on an expanding window to prevent look ahead bias
Beta neutral – Daily heatmap YTD:
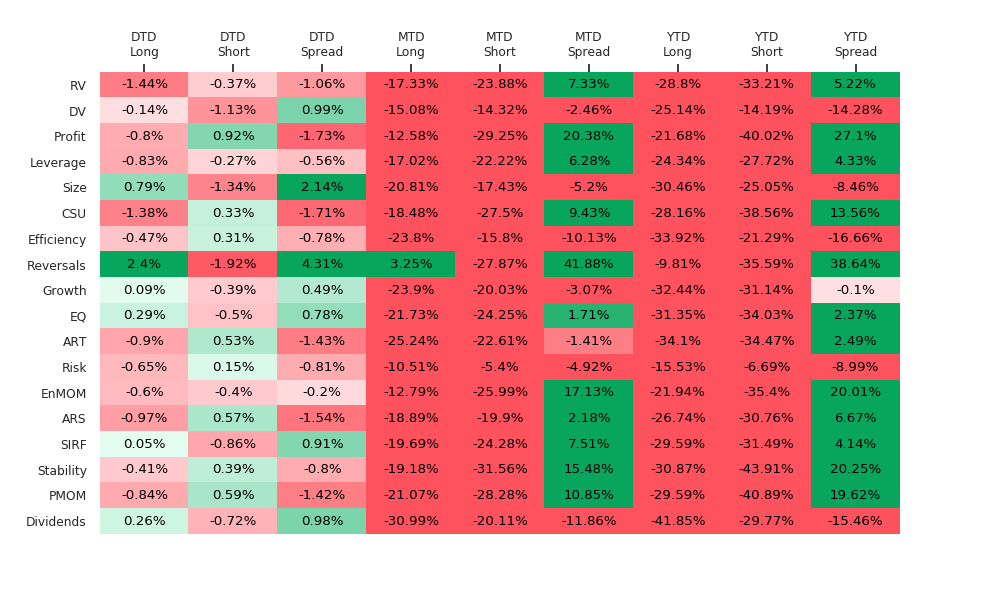
$ neutral – Daily heatmap YTD:
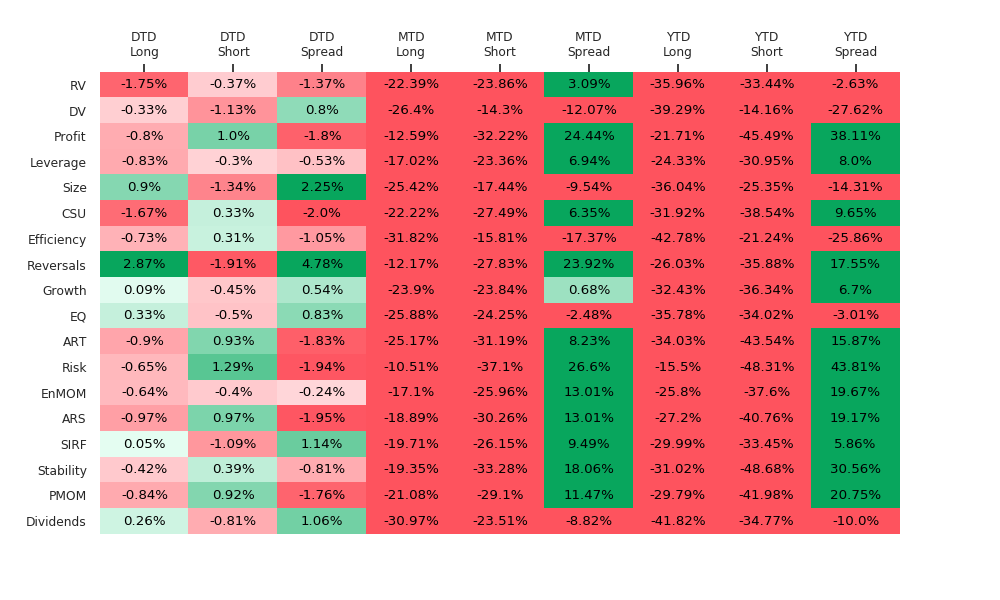
Beta-neutral – 19y Montly +1y Daily heatmap LTD:
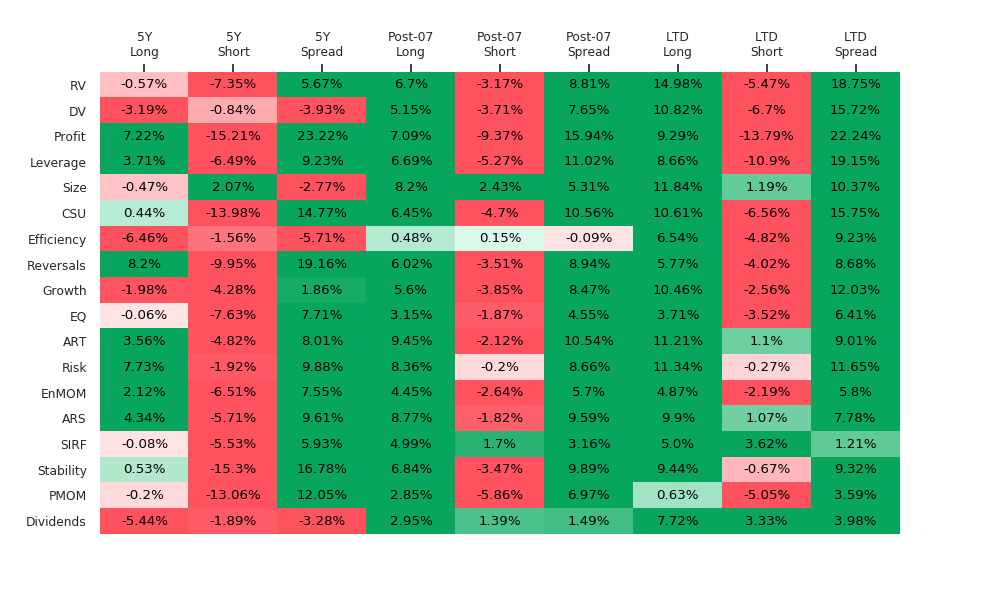
$ neutral – 19y Montly +1y Daily heatmap LTD:
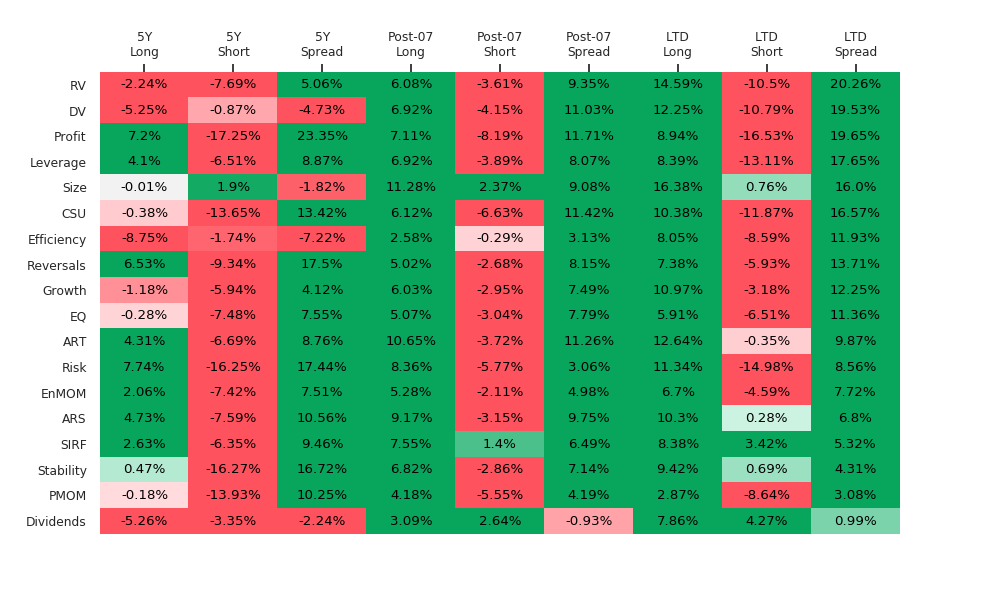
Sector ranks based on QMIT Enhanced Smart Betas:
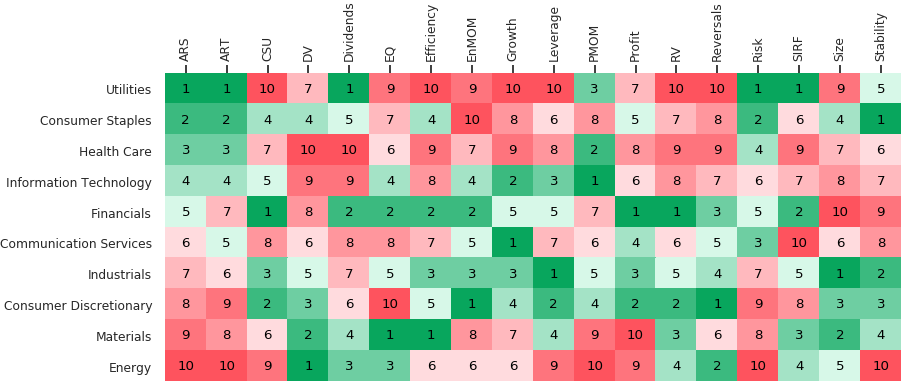
C-S Rank correlations for QMIT Enhanced Smart Betas:
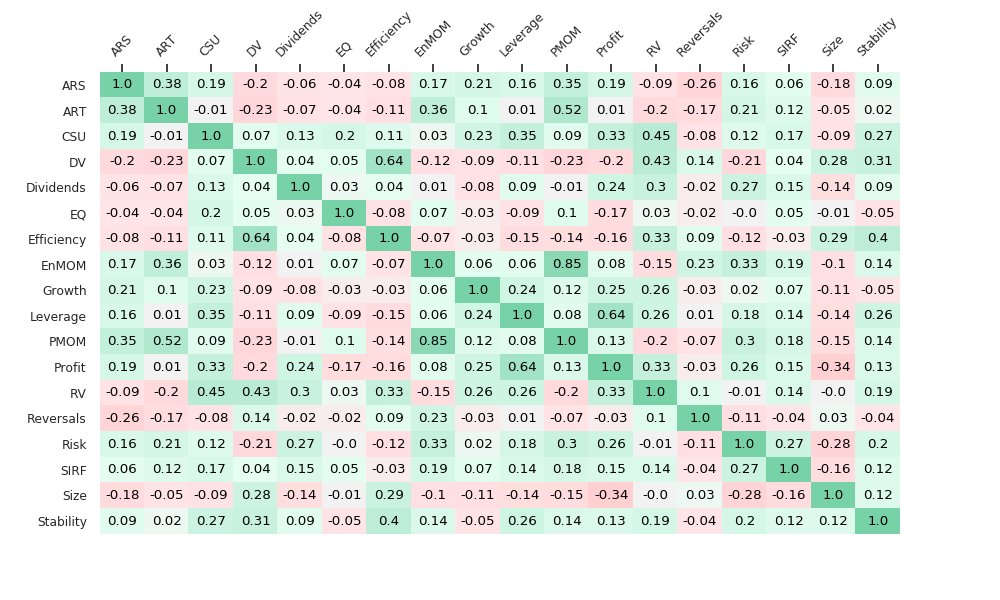
3y Return correlations for QMIT Enhanced Smart Betas:
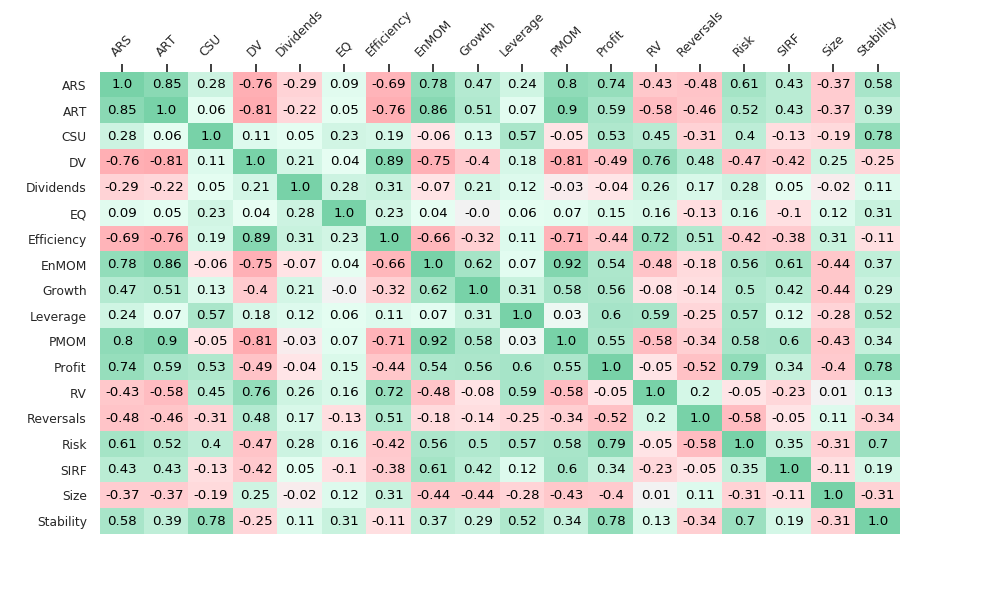
20y Return correlations for QMIT Enhanced Smart Betas:
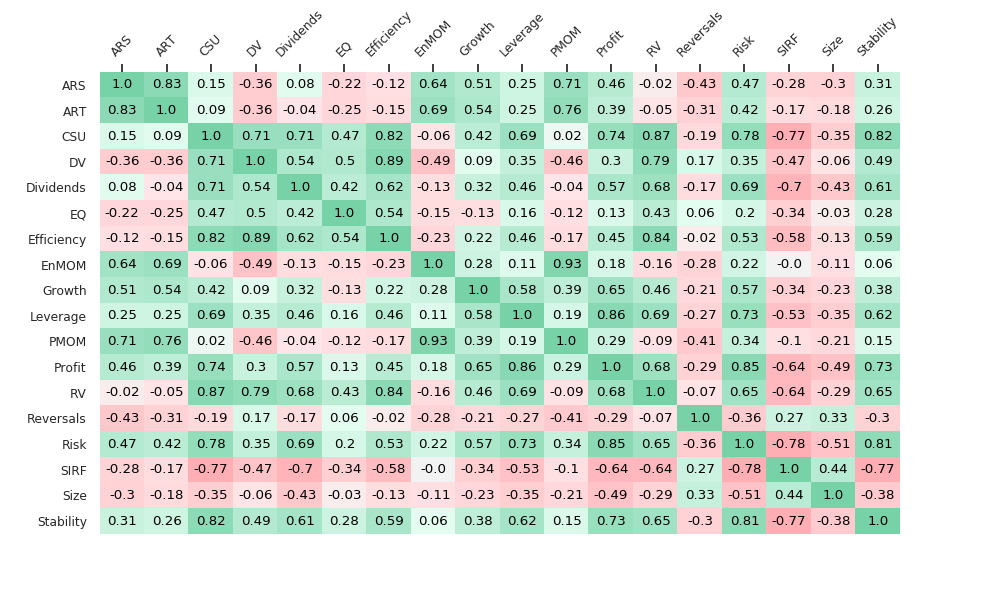
QMIT by QuantZ presents Q1 2020 Factor recap. To learn more about QuantZ/ QMIT and to get their factor research + heatmaps daily or even real-time, please get in touch!
EXPLANATORY FOOTNOTES:
Sector Ranks are aggregated bottom up average ranks for each of the smart beta composites.
Factor portfolios are not sector neutral.
Generated weekly as of last night’s close this report shows the DTD, MTD, YTD and LTD returns for our smart beta composite spreads.
Factors within the cohort spreads are long-short based on top vs bottom 5%-tile (~125×125) of the largest liquid US traded stocks (usually ~2500 depending upon market capitalization & minimum $ price criterion for stocks listed on NYSE & Nasdaq).
Certain industries like Biotechs and REITS are excluded due to event risk or because a generic quant model is not appropriate for those industries.
Individual factor top & bottom portfolios are equally weighted 5%-tiles. While the combined ESB spreads also represent top vs bottom 5%-tiles they are based on the best (cumulative return LTD) of five methodologies listed above.
MTD returns/ spreads are geometrically chain-linked DTD returns/ spreads where both are based on factor portfolios formed at the prior month end close.
YTD & LTD returns are based on geometric chain-linking of monthlies without transaction costs or fees as is customary in the factor literature.
Multi-period spread returns are not the difference of cumulative top vs bottom returns. Instead, they represent the daily geometrically compounded rebalancing of the market neutral “active return” differential of the top vs bottom portfolios which is a more realistic representation.
Both Max Sharpe & Risk Parity optimization routines are based on a Hybrid methodology where we 1] find the optimal factor mix within the Smart Beta cohort based on signal blending/ “mixing” but 2] subsequently run the combined ESB spreads outsample on a fully “integrated” basis not just as the linear combination of factor returns.
LTD data commences January 2000.
Enhanced Smart Beta Definitions
ARS: This smart beta composite shows our Analyst Revisions cohort based on measures of estimate revisions, dispersion, Standardized Unexpected Earnings surprise (SUE score) & consensus change in both earnings as well as revenues which can outperform traditional metrics like a 1mo consensus change.
ART: This smart beta composite shows our Analyst Ratings & Targets cohort based on measures of analyst recommendations, target price, changes & diffusion which can outperform traditional metrics like a 1mo consensus change.
CSU: This smart beta composite shows our Capital Structure/Usage cohort based on measures including Buybacks, Total yield, Capex, capital usage ratios etc which can outperform traditional metrics like Cash/MC.
Dividends: This smart beta composite shows our Dividends related cohort based on measures including Yield, payout, growth, forward yield etc which can outperform traditional metrics like Dividend Yield.
DV: This smart beta composite shows our Deep Value (or intrinsic value) cohort based on measures including tangible book & sales which can outperform traditional Book yield.
Efficiency: This smart beta composite shows our Efficiency cohort based on measures including Asset Turnover, Current Liabilities, Receivables etc which can outperform traditional metrics like Asset Turnover.
EnMOM: This smart beta composite shows our Enhanced Momentum cohort which can outperform traditional 12 month price momentum in both return & risk adjusted terms particularly at market inflection points.
EQ: This smart beta composite shows our Earnings Quality cohort based on a variety of Accrual measures which can outperform traditional metrics like Total Accruals.
Growth: This smart beta composite shows our Historical Growth cohort based on a variety of Earnings, Sales, Margins & CF related growth measures which can outperform traditional metrics like 3yr Sales growth.
Leverage: This smart beta composite shows our Leverage related cohort based on measures of Balance Sheet leverage which can outperform traditional metrics like Debt To Equity.
PMOM: This smart beta composite shows our PMOM related cohort which can outperform traditional 12 month price momentum using a variety of traditional momentum factors.
Profit: This smart beta composite shows our Profitability cohort based on measures like ROA, ROE, ROCE, ROTC, Margins etc which can outperform traditional metrics like ROE.
RV: This smart beta composite shows our Relative Value cohort based on measures of EPS, CFO, EBITDA etc which can outperform traditional Earnings yield.
Reversals: This smart beta composite shows our Reversals cohort which is comprised of metrics like short term reversals, RSI, DMA & other technical factors which can outperform traditional metrics like a 1 month total return.
Risk: This smart beta composite shows our Risk/ Low Vol cohort which is comprised of metrics like Beta, Low volatility etc.
SIRF: This smart beta composite shows our Short Interest cohort which is comprised of metrics related to Short Interest and its normalization by Float, trading volume etc.
Size: This smart beta composite shows our Size cohort which is comprised of metrics related to firm size including market capitalization.
Stability: This smart beta composite shows our Stability cohort which is comprised of metrics like Dispersion of EPS/ SPS estimates as well as the stability of Margins, EPS & CFs etc.
Disclosure: QMIT – QuantZ Machine Intelligence Technologies
QMIT is a data provider and not an investment advisor. This information has been prepared by QMIT for informational purposes only. This information should not be construed as investment, legal and/or tax advice. Additionally, this content is not intended as an offer to sell or a solicitation of any investment product or service. Opinions expressed are based on statistical forecasting from historical data. Past performance does not guarantee future performance. Further, the assumptions and the historical data based used could be erroneous. All results and analyses expressed are merely hypothetical and are NOT guaranteed. Trading securities involves substantial risk. Please consult a qualified investment advisor before risking any capital. The performance results for live portfolios following the screens presented herein may differ from the performance hypotheticals contained in this report for a variety of reasons, including differences related to transaction costs, market impact, fees, as well as differences in the time and price of execution. The performance results for individuals following the strategy could also differ based on differences in treatment of dividends received, including the amount received and whether and when such dividends were reinvested. We do not request personal information in any unsolicited email correspondence from our customers. Any correspondence offering trading advice or unsolicited message asking for personal details should be treated as fraudulent and reported to QMIT. Neither QMIT nor its third-party content providers shall be liable for any errors, inaccuracies or delays in content, or for any actions taken in reliance thereon. QMIT EXPRESSLY DISCLAIMS ALL WARRANTIES, EXPRESSED OR IMPLIED, AS TO THE ACCURACY OF ANY THE CONTENT PROVIDED, OR AS TO THE FITNESS OF THE INFORMATION FOR ANY PURPOSE. Although QMIT makes reasonable efforts to obtain reliable content from third parties, QMIT does not guarantee the accuracy of or endorse the views or opinions given by any third-party content provider. All content herein is owned by QuantZ Machine Intelligence Technologies and/ or its affiliates and protected by United States and international copyright laws. QMIT content may not be reproduced, transmitted or distributed without the prior written consent of QMIT.
Disclosure: Interactive Brokers Third Party
Information posted on IBKR Campus that is provided by third-parties does NOT constitute a recommendation that you should contract for the services of that third party. Third-party participants who contribute to IBKR Campus are independent of Interactive Brokers and Interactive Brokers does not make any representations or warranties concerning the services offered, their past or future performance, or the accuracy of the information provided by the third party. Past performance is no guarantee of future results.
This material is from QMIT – QuantZ Machine Intelligence Technologies and is being posted with its permission. The views expressed in this material are solely those of the author and/or QMIT – QuantZ Machine Intelligence Technologies and Interactive Brokers is not endorsing or recommending any investment or trading discussed in the material. This material is not and should not be construed as an offer to buy or sell any security. It should not be construed as research or investment advice or a recommendation to buy, sell or hold any security or commodity. This material does not and is not intended to take into account the particular financial conditions, investment objectives or requirements of individual customers. Before acting on this material, you should consider whether it is suitable for your particular circumstances and, as necessary, seek professional advice.
Join The Conversation
For specific platform feedback and suggestions, please submit it directly to our team using these instructions.
If you have an account-specific question or concern, please reach out to Client Services.
We encourage you to look through our FAQs before posting. Your question may already be covered!