Neural network studies were started in an effort to map the human brain and understand how humans make decisions but algorithms try to remove human emotions altogether from the trading aspect. What we sometimes fail to realise is that the human brain is quite possibly the most complex machine in this world and has been known to be quite effective at coming to conclusions in record time.
Think about it, if we could harness the way our brain works and apply it in the machine learning domain (neural networks are after all a subset of machine learning), we could possibly take a giant leap in terms of processing power and computing resources.
Before we dive deep into the nitty-gritty of neural network trading, we should understand the workings of the principal component, ie the neuron.
Remember, the end goal of the neural network tutorial is to understand the concepts involved in neural networks and how they can be applied to anticipate stock prices in the live markets. Let us start by understanding what a neuron is.
Structure of a Neuron
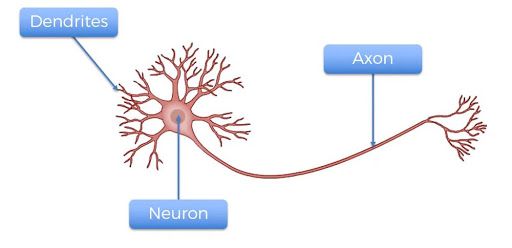
There are three components to a neuron, the dendrites, axon and the main body of the neuron. The dendrites are the receivers of the signal and the axon is the transmitter. Alone, a neuron is not of much use, but when it is connected to other neurons, it does several complicated computations and helps operate the most complicated machine on our planet, the human body.
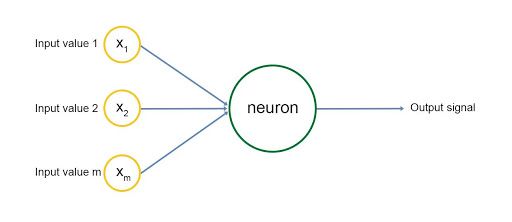
Perceptron: the Computer Neuron
A perceptron, ie a computer neuron, is built in a similar manner, as shown in the diagram.
There are inputs to the neuron marked with yellow circles, and the neuron emits an output signal after some computation.
The input layer resembles the dendrites of the neuron and the output signal is the axon. Each input signal is assigned a weight, wi. This weight is multiplied by the input value and the neuron stores the weighted sum of all the input variables. These weights are computed in the training phase of the neural network learning through concepts called gradient descent and backpropagation, we will cover these topics later on.
An activation function is then applied to the weighted sum, which results in the output signal of the neuron.
The input signals are generated by other neurons, i.e, the output of other neurons, and the network is built to make outlooks/computations in this manner.
This is the basic idea of a neural network. We will look at each of these concepts in more detail in this neural network tutorial.
Stay tuned for part II in which Devang will discuss how to understand a Neural Network.
Disclosure: Interactive Brokers Third Party
Information posted on IBKR Campus that is provided by third-parties does NOT constitute a recommendation that you should contract for the services of that third party. Third-party participants who contribute to IBKR Campus are independent of Interactive Brokers and Interactive Brokers does not make any representations or warranties concerning the services offered, their past or future performance, or the accuracy of the information provided by the third party. Past performance is no guarantee of future results.
This material is from QuantInsti and is being posted with its permission. The views expressed in this material are solely those of the author and/or QuantInsti and Interactive Brokers is not endorsing or recommending any investment or trading discussed in the material. This material is not and should not be construed as an offer to buy or sell any security. It should not be construed as research or investment advice or a recommendation to buy, sell or hold any security or commodity. This material does not and is not intended to take into account the particular financial conditions, investment objectives or requirements of individual customers. Before acting on this material, you should consider whether it is suitable for your particular circumstances and, as necessary, seek professional advice.
Join The Conversation
For specific platform feedback and suggestions, please submit it directly to our team using these instructions.
If you have an account-specific question or concern, please reach out to Client Services.
We encourage you to look through our FAQs before posting. Your question may already be covered!