See Part I, Part II , Part III, Part IV and Part V of this series to get started with the statistical terms and concepts used in Kalman Filter.
Pairs trading using Kalman Filter in Python
(Thanks to Chamundeswari Koppisetti for providing the code.)
Let us start by importing the necessary libraries for Kalman Filter
# Import a Kalman filter and other libraries
!pip install pykalman
!pip install qq-training-wheels auquan_toolbox –upgrade
from pykalman import KalmanFilter
import numpy as np
import pandas as pd
from scipy import poly1d
from datetime import datetime
import matplotlib.pyplot as plt
%matplotlib inline
plt.style.use(‘seaborn-darkgrid’)
plt.rcParams[‘figure.figsize’] = (10,7)
We will consider the 4 year (Aug 2015 – Aug 2019) Adjusted Close price data for Bajaj Auto Limited (BAJAJ-AUTO.NS) and Hero MotoCorp Limited (HEROMOTOCO.NS).
We have included the data file in the zip file along with the code for you to run on your system later. The link to download the files can be found at the end of the blog.
# Define path where data file is saved in your system
#path = ‘../data/’
data = pd.read_csv(path +’data.csv’, index_col =’Date’)
data[‘ratio’] = data[‘BAJAJ’]/ data[‘HERO’]
stock_1 = data[‘BAJAJ’]
stock_2 = data[‘HERO’]
# Calculate the hedge ratio for pairs trading
ratio =stock_1/stock_2
data.head()
The output will be as follows:
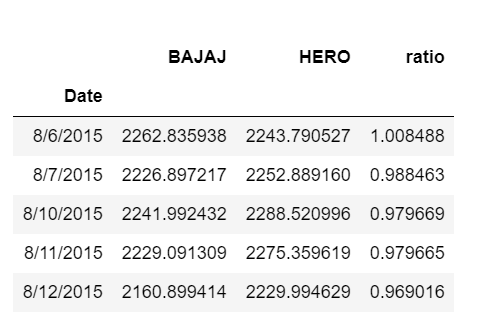
Hyperparameters of Kalman Filter can be changed for instance:
- Multi dimensional transition matrices, to use more of past information for making expected results at each point
- Different values of observation and transition covariance
kf = KalmanFilter(transition_matrices = [1],
observation_matrices = [1],
initial_state_mean = 0,
initial_state_covariance = 1,
observation_covariance=1,
transition_covariance=.0001)
mean, cov = kf.filter(ratio.values)
mean, std = mean.squeeze(), np.std(cov.squeeze())
plt.figure(figsize=(15,7))
plt.plot(ratio.values – mean, ‘m’, lw=1)
plt.plot(np.sqrt(cov.squeeze()), ‘y’, lw=1)
plt.plot(-np.sqrt(cov.squeeze()), ‘c’, lw=1)
plt.title(‘Kalman filter estimate’)
plt.legend([‘Error: real_value – mean’, ‘std’, ‘-std’])
plt.xlabel(‘Day’)
plt.ylabel(‘Value’)
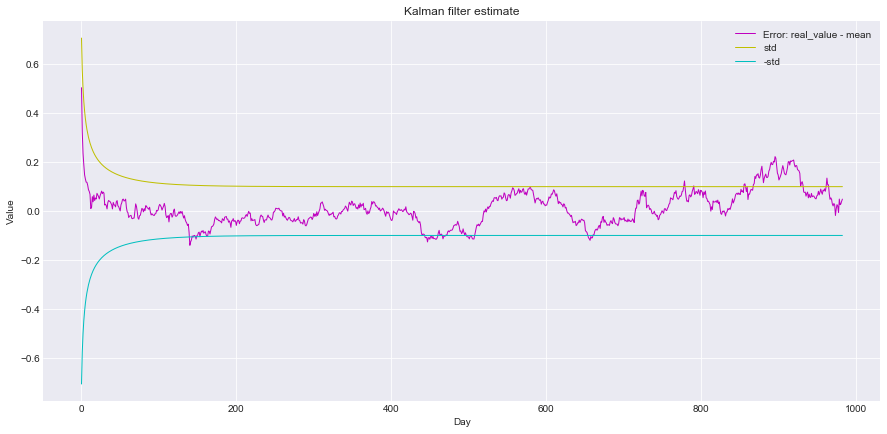
Stay tuned for the next installment, in which Rekhit will showcase how to use Python for a Pairs trading strategy script.
Download the full code: https://blog.quantinsti.com/kalman-filter/.
All data and information provided in this article are for informational purposes only. QuantInsti® makes no representations as to accuracy, completeness, currentness, suitability, or validity of any information in this article and will not be liable for any errors, omissions, or delays in this information or any losses, injuries, or damages arising from its display or use. All information is provided on an as-is basis.
Disclosure: Interactive Brokers Third Party
Information posted on IBKR Campus that is provided by third-parties does NOT constitute a recommendation that you should contract for the services of that third party. Third-party participants who contribute to IBKR Campus are independent of Interactive Brokers and Interactive Brokers does not make any representations or warranties concerning the services offered, their past or future performance, or the accuracy of the information provided by the third party. Past performance is no guarantee of future results.
This material is from QuantInsti and is being posted with its permission. The views expressed in this material are solely those of the author and/or QuantInsti and Interactive Brokers is not endorsing or recommending any investment or trading discussed in the material. This material is not and should not be construed as an offer to buy or sell any security. It should not be construed as research or investment advice or a recommendation to buy, sell or hold any security or commodity. This material does not and is not intended to take into account the particular financial conditions, investment objectives or requirements of individual customers. Before acting on this material, you should consider whether it is suitable for your particular circumstances and, as necessary, seek professional advice.
Disclosure: Displaying Symbols on Video
Any stock, options or futures symbols displayed are for illustrative purposes only and are not intended to portray recommendations.
Join The Conversation
For specific platform feedback and suggestions, please submit it directly to our team using these instructions.
If you have an account-specific question or concern, please reach out to Client Services.
We encourage you to look through our FAQs before posting. Your question may already be covered!